Division of Environment and Natural Resources
SINOGRAIN II
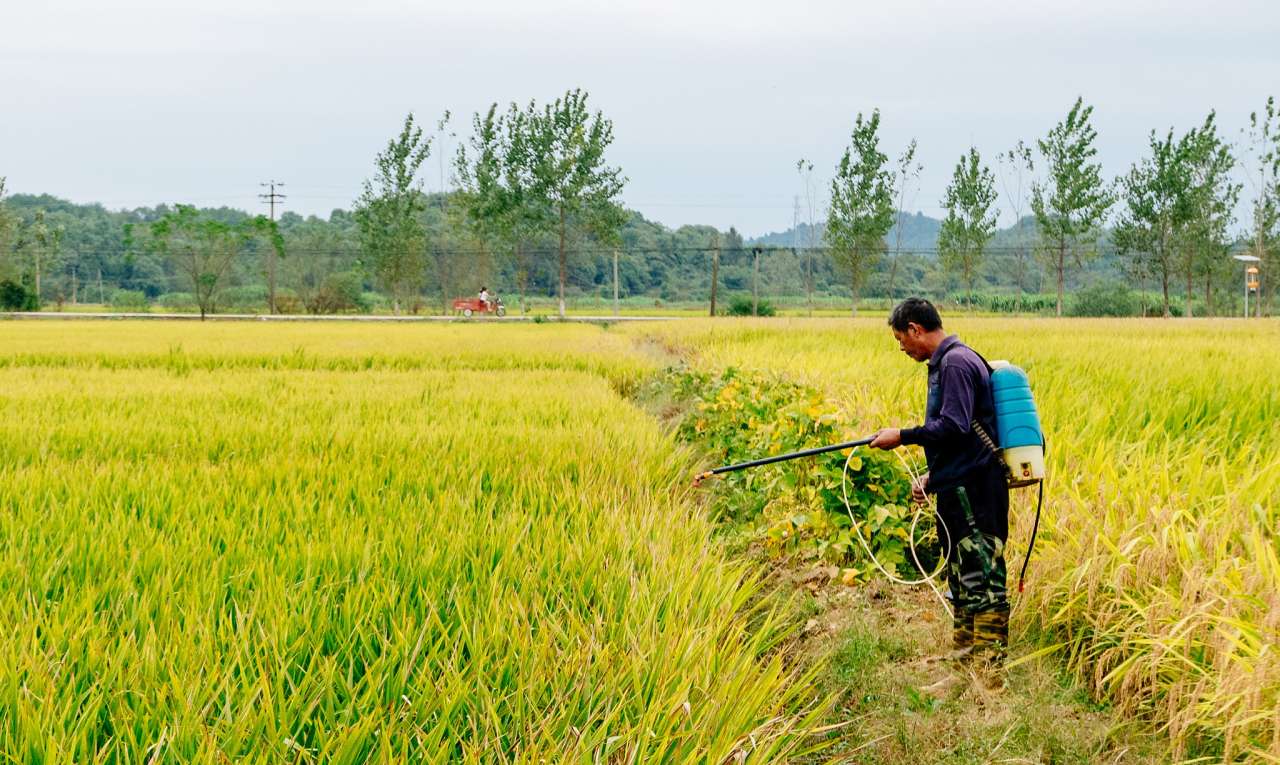
End: sep 2023
Start: oct 2018
Scientists from NIBIO and CAAS are working together using innovative technologies in order to improve productivity, food safety and sustainability in Chinese agriculture.
More information
Sinograin IIProject participants
Berit Nordskog Marianne Stenrød Hege Særvold Steen Nicholas Clarke Tor-Einar Skog Krzysztof Kusnierek Xingang Liu Deliang Peng Siri Elise Dybdal Yuxin Miao Jiangsan Zhao Baozhong Hu Xiande Li Zhou Xueping Paulsen Mariann Erling Fløistad Knut Øistad Giovanna Ottaviani Aalmo Divina Gracia P. Rodriguez Einar Strand Igor A. Yakovlev Inger Heldal Andre van EerdeStatus | Active |
Start - end date | 01.10.2018 - 30.09.2023 |
Project manager | Jihong Liu Clarke |
Division | Division of Environment and Natural Resources |
Department | Biogeochemistry and Soil Quality |
Partners | Chinese Academy of Agricultural Sciences (CAAS), China Agriculture University (CAU, Heilongjiang Academy of Agricultural Sciences (HAAS), Harbin University (HAU), China National Rice Research Institute (CNRRI), Xinjiang Academy of Agricultural Sciences (XAAS). |
Total budget | NOK 20,2 mill |
Globally demand for food is growing while arable land is declining. The UN predicts global food demand will increase by 60% by 2050. However, only 20% of new food production is expected to come from expansion of new farming land.
A key to meeting the growing food demands will be a global shift towards more sustainable agricultural systems that reduce environmental footprints at the same time as increasing food production.
Precision farming and reduction of fertilizer and pesticide applications are two technologies that have proven to have great environmental and economic benefits in agricultural production in Norway.
Scientists from NIBIO, the Chinese Academy for Agricultural Sciences (CAAS) and other Chinese partners will work together in a four-year project (Sinograin II) funded by the Norwegian Ministry of Foreign Affairs through the Royal Norwegian Embassy in Beijing, using such innovative technologies in an effort to improve productivity, food safety and sustainability in Chinese agriculture.
The project is a step forward from the work done in the project Sinograin I, and one of the several ongoing collaborative projects between NIBIO and CAAS in China focusing on climate smart and sustainable food production.
Financing details
The Ministry of Foreign Affairs contributes 18.8 million of a total budget of 20.2 million NOK.
In addition to Sinograin II, NIBIO and the Chinese partners, especially CAAS, have received four other grants from EU-China H2020, RCN (Research Council of Norway) - NSFC (National Natural Science Foundation of China) joint calls and The Ministry of Agriculture and Food in Norway.
This has a total funding of approximately 68-70 million NOK for a period of 3-4 years (2018-2022).
Around 40 NIBIO staff is working on those projects.
Poster SINOGRAIN II
Report from Consultative Meeting on Fall Armyworm in Asia
Forebygging av eventuell global matsikkerhetskrise forårsaket av Covid-19-pandemien
Article written by Prof. Xiande Li, CAAS. Translated to Norwegian by Research Prof. Jihong Liu Clarke, NIBIO
Original Article written by Prof. Xiande Li, CAAS and published in Farmers's Daily, China
Article in Chineese
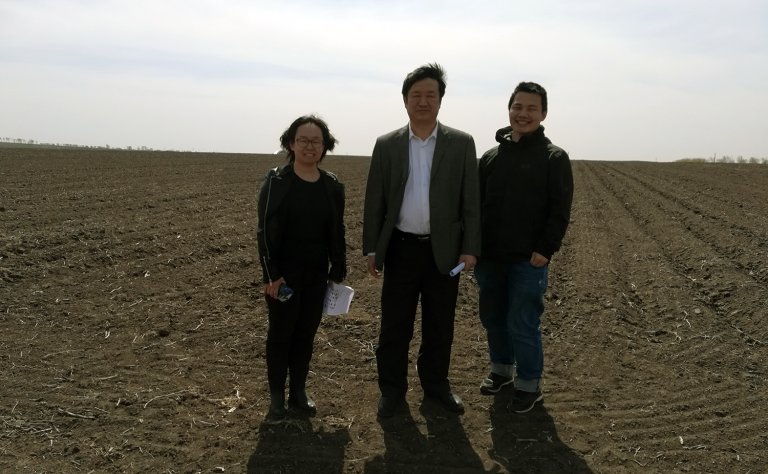
Publications in the project
Authors
Xinbing Wang Yuxin Miao Rui Dong Hainie Zha Tingting Xia Zhichao Chen Krzysztof Kusnierek Guohua Mi Hong Sun Minzan LiAbstract
Reliable and efficient in-season nitrogen (N) status diagnosis and recommendation methods are crucially important for the success of crop precision N management (PNM). The accuracy of these methods has been found to be influenced by soil properties, weather conditions, and crop management practices. It is important to effectively incorporate these variables to improve in-season N management. Machine learning (ML) methods are promising due to their capability of processing different types of data and modeling both linear and non-linear relationships. The objectives of this study were to (1) determine the potential improvement of in-season prediction of corn N nutrition index (NNI) and grain yield by combining soil, weather and management data with active sensor data using random forest regression (RFR) as compared with Lasso linear regression (LR) using similar data and simple regression (SR) models only using crop sensor data; and (2) to develop a new in-season side-dress N fertilizer recommendation strategy at eighth to ninth leaf stage (V8-V9) of corn developement using the RFR model. Twelve site-year experiments examining corn N rates and planting densities were conducted in Northeast China. The GreenSeeker sensor data and corn NNI were collected at V8-V9 stage, and grain yield was determined at the harvest stage (R6). The soil information was obtained at planting and the weather data was measured throughout the growing season. The results indicated that corn NNI and grain yield were better predicted by combining soil, weather and management information with GreenSeeker sensor data using RFR model (R2 = 0.86 and 0.79) and LR model (R2 = 0.85 and 0.76) as compared with only using GreenSeeker sensor data (R2 = 0.66 and 0.62–63) based on the test dataset. An innovative in-season side-dress N recommendation strategy was developed using the RFR grain yield prediction model to simulate corn grain yield responses to a series of side-dress N rates at V8-V9 stage. Based on these response curves, site-, and year-specific optimum side-dress N rates can be determined. The scenario analysis results indicated that this RFR model-based in-season N recommendation strategy could recommend side-dress N rates similar to those based on measured agronomic optimum N rate (AONR) or economic optimum N rate (EONR), with root mean square error (RMSE) of 17 kg ha−1 and relative error (RE) of 14–15 %. It is concluded that combining soil, weather and management information with crop sensor data using RFR can significantly improve both in-season corn NNI and grain yield prediction and N management, compared with the approach based only on crop sensor data. More studies are needed to further improve and evaluate this approach under diverse on-farm conditions.
Authors
Hainie Zha Yuxin Miao Tiantian Wang Yue Li Jing Zhang Weichao Sun Zhengqi Feng Krzysztof KusnierekAbstract
Optimizing nitrogen (N) management in rice is crucial for China’s food security and sustainable agricultural development. Nondestructive crop growth monitoring based on remote sensing technologies can accurately assess crop N status, which may be used to guide the in-season site-specific N recommendations. The fixed-wing unmanned aerial vehicle (UAV)-based remote sensing is a low-cost, easy-to-operate technology for collecting spectral reflectance imagery, an important data source for precision N management. The relationships between many vegetation indices (VIs) derived from spectral reflectance data and crop parameters are known to be nonlinear. As a result, nonlinear machine learning methods have the potential to improve the estimation accuracy. The objective of this study was to evaluate five different approaches for estimating rice (Oryza sativa L.) aboveground biomass (AGB), plant N uptake (PNU), and N nutrition index (NNI) at stem elongation (SE) and heading (HD) stages in Northeast China: (1) single VI (SVI); (2) stepwise multiple linear regression (SMLR); (3) random forest (RF); (4) support vector machine (SVM); and (5) artificial neural networks (ANN) regression. The results indicated that machine learning methods improved the NNI estimation compared to VI-SLR and SMLR methods. The RF algorithm performed the best for estimating NNI (R2 = 0.94 (SE) and 0.96 (HD) for calibration and 0.61 (SE) and 0.79 (HD) for validation). The root mean square errors (RMSEs) were 0.09, and the relative errors were <10% in all the models. It is concluded that the RF machine learning regression can significantly improve the estimation of rice N status using UAV remote sensing. The application machine learning methods offers a new opportunity to better use remote sensing data for monitoring crop growth conditions and guiding precision crop management. More studies are needed to further improve these machine learning-based models by combining both remote sensing data and other related soil, weather, and management information for applications in precision N and crop management.
Abstract
The dynamic interactions between soil, weather and crop management have considerable influences on crop yield within a region, and should be considered in optimizing nitrogen (N) management. The objectives of this study were to determine the influence of soil type, weather conditions and planting density on economic optimal N rate (EONR), and to evaluate the potential benefits of site-specific N management strategies for maize production. The experiments were conducted in two soil types (black and aeolian sandy soils) from 2015 to 2017, involving different N rates (0 to 300 kg ha−1) with three planting densities (55,000, 70,000, and 85,000 plant ha−1) in Northeast China. The results showed that the average EONR was higher in black soil (265 kg ha−1) than in aeolian sandy soil (186 kg ha−1). Conversely, EONR showed higher variability in aeolian sandy soil (coefficient of variation (CV) = 30%) than in black soil (CV = 10%) across different weather conditions and planting densities. Compared with farmer N rate (FNR), applying soil-specific EONR (SS-EONR), soil- and year-specific EONR (SYS-EONR) and soil-, year-, and planting density-specific EONR (SYDS-EONR) would significantly reduce N rate by 25%, 30% and 38%, increase net return (NR) by 155 $ ha−1, 176 $ ha−1, and 163 $ ha−1, and improve N use efficiency (NUE) by 37–42%, 52%, and 67–71% across site-years, respectively. Compared with regional optimal N rate (RONR), applying SS-EONR, SYS-EONR and SYDS-EONR would significantly reduce N application rate by 6%, 12%, and 22%, while increasing NUE by 7–8%, 16–19% and 28–34% without significantly affecting yield or NR, respectively. It is concluded that soil-specific N management has the potential to improve maize NUE compared with both farmer practice and regional optimal N management in Northeast China, especially when each year’s weather condition and planting density information is also considered. More studies are needed to develop practical in-season soil (site)-specific N management strategies using crop sensing and modeling technologies to better account for soil, weather and planting density variation under diverse on-farm conditions.
Authors
Jiangsan Zhao Dmitry Kechasov Boris Rewald Gernot Bodner Michel Verheul Nicholas Clarke Jihong Liu ClarkeAbstract
Hyperspectral imaging has many applications. However, the high device costs and low hyperspectral image resolution are major obstacles limiting its wider application in agriculture and other fields. Hyperspectral image reconstruction from a single RGB image fully addresses these two problems. The robust HSCNN-R model with mean relative absolute error loss function and evaluated by the Mean Relative Absolute Error metric was selected through permutation tests from models with combinations of loss functions and evaluation metrics, using tomato as a case study. Hyperspectral images were subsequently reconstructed from single tomato RGB images taken by a smartphone camera. The reconstructed images were used to predict tomato quality properties such as the ratio of soluble solid content to total titratable acidity and normalized anthocyanin index. Both predicted parameters showed very good agreement with corresponding “ground truth” values and high significance in an F test. This study showed the suitability of hyperspectral image reconstruction from single RGB images for fruit quality control purposes, underpinning the potential of the technology—recovering hyperspectral properties in high resolution—for real-world, real time monitoring applications in agriculture any beyond.
Authors
Zhichao Chen Yuxin Miao Junjun Lu Lan Zhou Yue Li Hongyan Zhang Weidong Lou Zheng Zhang Krzysztof Kusnierek Changhua LiuAbstract
Improving nitrogen (N) management of small-scale farming systems in developing countries is crucially important for food security and sustainable development of world agriculture, but it is also very challenging. The N Nutrition Index (NNI) is a reliable indicator for crop N status, and there is an urgent need to develop an effective method to non-destructively estimate crop NNI in different smallholder farmer fields to guide in-season N management. The eBee fixed-wing unmanned aerial vehicle (UAV)-based remote sensing system, a ready-to-deploy aircraft with a Parrot Sequoia+ multispectral camera onboard, has been used for applications in precision agriculture. The objectives of this study were to (i) determine the potential of using fixed-wing UAV-based multispectral remote sensing for non-destructive estimation of winter wheat NNI in different smallholder farmer fields across the study village in the North China Plain (NCP) and (ii) develop a practical strategy for village-scale winter wheat N status diagnosis in small scale farming systems. Four plot experiments were conducted within farmer fields in 2016 and 2017 in a village of Laoling County, Shandong Province in the NCP for evaluation of a published critical N dilution curve and for serving as reference plots. UAV remote sensing images were collected from all the fields across the village in 2017 and 2018. About 150 plant samples were collected from farmer fields and plot experiments each year for ground truthing. Two indirect and two direct approaches were evaluated for estimating NNI using vegetation indices (VIs). To facilitate practical applications, the performance of three commonly used normalized difference VIs were compared with the top performing VIs selected from 59 tested indices. The most practical and stable method was using VIs to calculate N sufficiency index (NSI) and then to estimate NNI non-destructively (R2 = 0.53–0.56). Using NSI thresholds to diagnose N status directly was quite stable, with a 57–59% diagnostic accuracy rate. This strategy is practical and least affected by the choice of VIs across fields, varieties, and years. This study demonstrates that fixed-wing UAV–based remote sensing is a promising technology for in-season diagnosis of winter wheat N status in smallholder farmer fields at village scale. The considerable variability in local soil conditions and crop management practices influenced the overall accuracy of N diagnosis, so more studies are needed to further validate and optimize the reported strategy and consecutively develop practical UAV remote sensing–based in-season N recommendation methods.
Authors
Ning Wang Huan Peng Shi-ming Liu Wen-kun Huang Ricardo Holgado Jihong Liu Clarke De-liang PengAbstract
Soybean cyst nematode (SCN, Heterodera glycines (I.)) is one of the most important soil-borne pathogens for soybeans. In plant parasitic nematodes, including SCN, lysozyme plays important roles in the innate defense system. In this study, two new lysozyme genes (Hg-lys1 and Hg-lys2) from SCN were cloned and characterized. The in situ hybridization analyses indicated that the transcripts of both Hg-lys1 and Hg-lys2 accumulated in the intestine of SCN. The qRT-PCR analyses showed that both Hg-lys1 and Hg-lys2 were upregulated after SCN second stage juveniles (J2s) were exposed to the Gram-positive bacteria Bacillus thuringiensis, Bacillus subtilis or Staphylococcus aureus. Knockdown of the identified lysozyme genes by in vitro RNA interference caused a significant decrease in the survival rate of SCN. All of the obtained results indicate that lysozyme is very important in the defense system and survival of SCN.