Jonathan Rizzi
Research Scientist
Attachments
List of PublicationsBiography
The main research activities are concerned with the use of GIS in several environmental sectors, including climate change, contaminated sites and water quality. Development of GIS-based tools such as a Spatial Decision Support System for climate change impact assessment (DESYCO) and WebGIS for climate data. He also worked on the definition of methodologies addressing climate change impacts of coastal zones useful to support the definition of adaptation measures and he has experience in MultiCriteria Decision Analysis (MCDA).
In the last years, he has also participated and managed international cooperation projects in developing countries.
Abstract
Urban green structures (UGS) play important roles in enhancing urban ecosystems by providing benefits such as mitigating the urban heat island effect, improving air quality, supporting biodiversity, and aiding in stormwater management. Accurately mapping UGS is important for sustainable urban planning and management. Traditional methods of mapping such as manual mapping, aerial photography interpretation and pixel-based classification have limitations in terms of coverage, accuracy, and efficiency. Object-based image analysis (OBIA) has gained prominence due to its ability to incorporate both spectral and spatial information making it particularly effective for classification of high-resolution satellite data. This paper reviews the application of OBIA on satellite images for UGS mapping, focusing on various data sources, popular segmentation methods, and classification techniques, highlighting their respective advantages and limitations. Key segmentation methodologies discussed include multi-resolution segmentation and watershed segmentation. For classification, the review covers machine learning techniques such as random forests, support vector machines, and convolutional neural networks, among others. Several case studies highlight the successful implementation of OBIA in diverse urban environments by demonstrating improvements in classification accuracy and detail. The review also addresses the challenges associated with OBIA, such as dealing with heterogenous urban landscapes, data sources and with OBIA methods itself. Future directions for UGS mapping include the integration of deep learning algorithms, advancements in satellite data technologies, and the development of standardized classification frameworks. By providing a detailed analysis of the current state-of-the-art in object-based UGS mapping, this review aims to guide future research and practical applications in UGS management.
Authors
Belachew Gizachew Zeleke Deo D. Shirima Jonathan Rizzi Collins Byobona Kukunda Eliakimu ZahabuAbstract
Tanzania dedicates a substantial proportion (38%) of its territory to conservation, with a large number of Protected Areas (PAs) managed under various regimes. Nevertheless, the country still experiences high rates of deforestation, which threaten the ecological integrity and socio-economic benefits of its forests. We utilized the Global Forest Change Dataset (2012–2022) and implemented a Propensity Score Matching (PSM) approach followed by a series of binomial logit regression modeling. Our objectives were to evaluate (1) the likelihood of PAs in avoiding deforestation compared with unprotected forest landscapes, (2) the variability in effectiveness among the different PA management regimes in avoiding deforestation, (3) evidence of leakage, defined here as the displacement of deforestation beyond PA boundaries as a result of protection inside PAs. Our findings reveal that, despite ongoing deforestation within and outside of PAs, conservation efforts are, on average, three times more likely to avoid deforestation compared with unprotected landscapes. However, the effectiveness of avoiding deforestation significantly varies among the different management regimes. National Parks and Game Reserves are nearly ten times more successful in avoiding deforestation, likely because of the stringent set of regulations and availability of resources for implementation. Conversely, Nature Forest Reserves, Game Controlled Areas, and Forest Reserves are, on average, only twice as likely to avoid deforestation, indicating substantial room for improvement. We found little evidence of the overall leakage as a consequence of protection. These results highlight the mixed success of Tanzania’s conservation efforts, suggesting opportunities to enhance the effectiveness of many less protected PAs. We conclude by proposing potential strategic pathways to enhance further the climate and ecosystem benefits of conservation in Tanzania.
Authors
Heine Nygard Riise Erlend Hustad Honningdalsnes Dagmar Hagen Markus A. K. Sydenham Anne Catriona Melhoop Trond Aalvik Simensen Jonathan Rizzi Torunn KjeldstadAbstract
No abstract has been registered
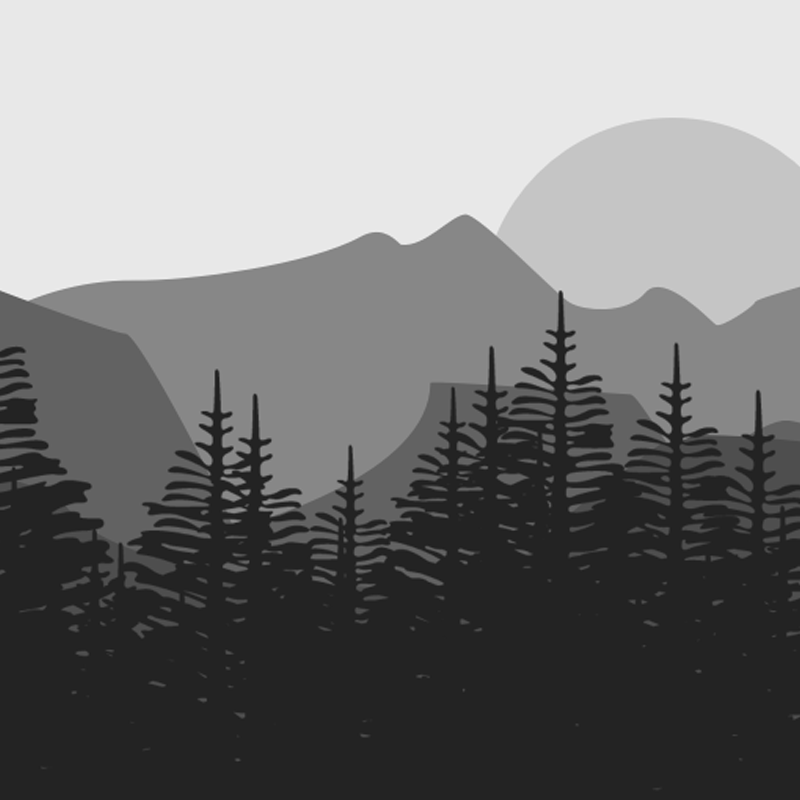
Division of Survey and Statistics
Pan-European Network of Green Deal Agriculture and Forestry Earth Observation Science (PANGEOS)
The sustainability of Europe’s green resources are threatened by climate change associated environmental changes. Agricultural systems and forests are among the ecosystems mostly interlinked with human health and wellbeing due to the socio-economic services they provide.
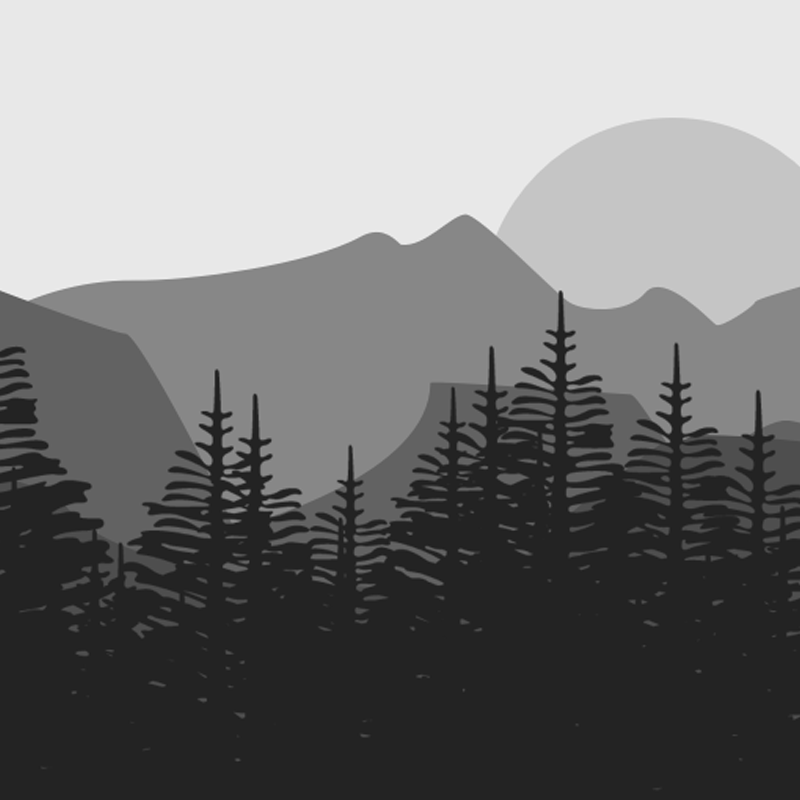
Division of Survey and Statistics
Considering the Environment and Nature when Building and Operating Ground Mounted Solar Power Plants in Norway
EnviSol's mission is to harmonize the growth of ground-mounted solar power plants in Norway with the imperative to protect biodiversity and ecosystem services. With renewable energy production, preserving nature, and supporting ecosystems all in mind, EnviSol aims to pinpoint the ideal methods and locations for these solar installations, mitigating clashes over land use.
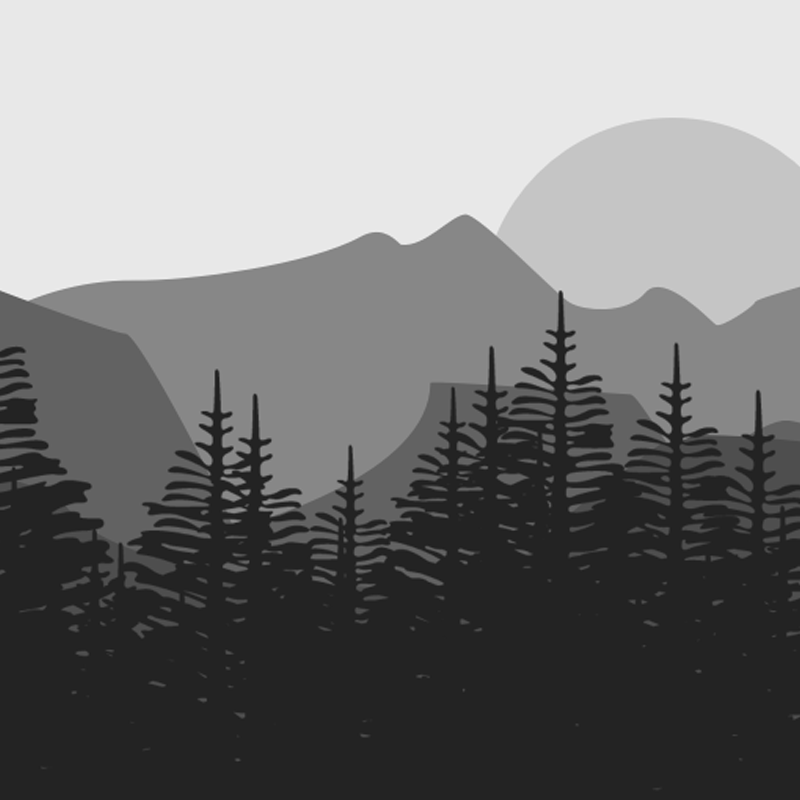
Division of Environment and Natural Resources
CANALLS Agroecological practices for sustainable transition
Agroecology covers all activities and actors involved in food systems. It also places the well-being of people (producers and consumers of crops and products) at its core. The EU-funded CANALLS project will focus on the agroecological zones and diverse farming systems in the humid tropics of Central and Eastern Africa. It will explore the complex environmental, social and economic challenges, which in some cases are exacerbated by conflict and high vulnerability. Moreover, it will advance agroecological transitions in these regions through multi-actor transdisciplinary agroecology Living Labs at eight sites in four countries. The focus will be on crops such as cocoa, coffee and cassava, which are vital for subsistence and economic development.
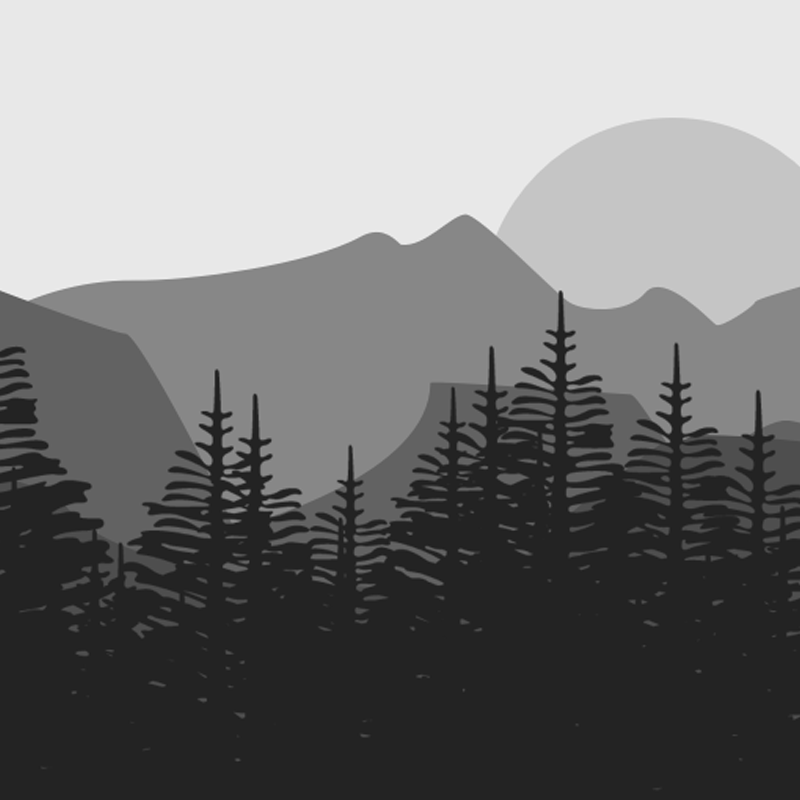
Division of Food Production and Society
Sustainable growth of the Norwegian Horticulture Food System – GreenRoad GS35 (“GrøntStrategi mot 2035)
The main aim of GreenRoad is to deliver knowledge and solutions for increased value creation and sustainability in the horticultural food system in Norway.